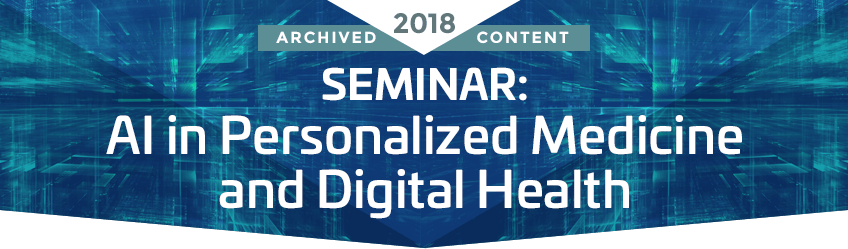
Monday, December 3, 2018 | 1:15 - 5:00 pm
AI aims to move medical treatment away from general, one-size-fits-all options to more personalized solutions. Learn more about the incredible amounts of information generated through precision medicine, and the critical capabilities needed to analyse,
store, normalize and trace that data.
1:15 Seminar Chair:
Kamal Jethwani, MD, Senior Director, Connected Health Innovation,
Partners HealthCare (PHS), Assistant Professor, Harvard Medical School (HMS)
1:20 Personalized Medicine: A Vision for Research and Education
Dimitris Bertsimas, PhD, Operations Research Center
and Sloan School of Management, MIT
Medicine as taught in medical schools and as practiced today is not personalized. We present a research program to develop an algorithmic theory of personalized medicine applied to the major human diseases. Using electronic medical records and genomic
data and in collaboration with major medical centers and medical doctors, we present our ongoing efforts to develop algorithms that propose personalized treatments for individual patients that suffer from these diseases. The new personalized medicine
methods are based on new cutting-edge Machine Learning algorithms that use modern optimization methods and improve upon classical approaches. We report on our work on diabetes, obesity and cardiovascular disease. We further discuss the implications
of our work on the education of the next generation of doctors.
1:50 Machine Learning Based Patient Subgroup Identification for Precision Medicine
Jie Cheng, PhD, Director, Data & Statistical Sciences, AbbVie
Central to precision medicine is the ability to effectively identify patient subgroups that are likely to benefit from a certain treatment. However, due to multiple testing, subgroup identification is well known for its tendency to produce spurious results
if not done with extreme care. In this talk, we will present a machine learning based patient subgroup identification approach and demonstrate its effectiveness using randomized clinical trial datasets.
2:20 Networking Break
2:50 Digital Health: The Use of Machine Learning and Deep Learning Techniques to Identify Patients and to Personalise Interventions
Kamal Jethwani, MD, Senior Director, Connected Health Innovation,
Partners HealthCare (PHS); Assistant Professor, Harvard Medical School (HMS)
The enormous amounts of data our healthcare systems hold can powerfully assist us in understanding which patients need help, and what type of help. AI can unlock this power by helping us stratify patients based on their risk for poor health outcomes,
and connecting them to interventions that would be most meaningful to them. Learn about risk stratification, digital biomarkers, and strategies to design engaging digital health tools using AI.
3:10 AI-Enabled Digital Biomarker Development
Wei-Yi Cheng, PhD, Senior Data Scientist, Roche
Digitalization has enabled innovative ways to understand neurological diseases. Smartphone-based remote monitoring poses as a potential solution to provide long-term, objective assessment of gait and mobility in patients with motor disorders such
as Parkinson’s Disease. Using Deep Learning, we successfully translated the sensor data collected by mobile phones into meaningful mobility information. We will also discuss some other potential applications of Deep Learning to help drug
development.
3:30 Introducing New Standards for Patient Care: The Role of AI in Precision Oncology
Amélie Boichard, PharmD, PhD, Project Scientist,
Center for Personalized Cancer Therapy, UCSD Moores Cancer Center
The development of high-throughput molecular analyses and specifically targeted anti-cancer drugs leads to new expectations for cancer patients that can now receive personalized and optimized treatment options, potentially demonstrating favourable
outcomes. Big data are a major driver in the development of Precision Oncology, and efficient analysis methods are needed to transform numerous information sources into actionable knowledge. Integrating algorithmic-generated techniques into decision-making
will soon advance the standard-of-care in oncology practice.
3:50 Reduction of High-Dimensional Genomic RNA-seq Data to Lower Dimensional Latent Space by Deep Learning
Shanrong Zhao, PhD, Director, Computational Biology, Pfizer
Biology is complex, consisting of multiple nonlinear and often redundant connections among genes, and when a specific pathway aberration occurs, the downstream response to the perturbation is captured in the transcriptome. The VAE (Variatonal autoencoder)
and GANs (Generative Adversarial Networks) can discover nonlinear explanatory features and have emerged as powerful deep leaning methods for reduction of high-dimensional RNA-seq data into lower dimensional latent space.
4:15 PANEL: AI in Personalized Medicine and Digital Health
Moderator:
Amélie Boichard, PharmD, PhD, Project Scientist,
Center for Personalized Cancer Therapy, UCSD Moores Cancer Center
Panelists:
Dimitris Bertsimas, PhD, Operations Research
Center and Sloan School of Management, MIT
Jie Cheng, PhD, Associate Director, Data & Statistical Sciences,
Abbvie
Danielle Ciofani, Director, Data Strategy and
Alliances, Broad Institute of MIT and Harvard
5:00 Close of Seminar