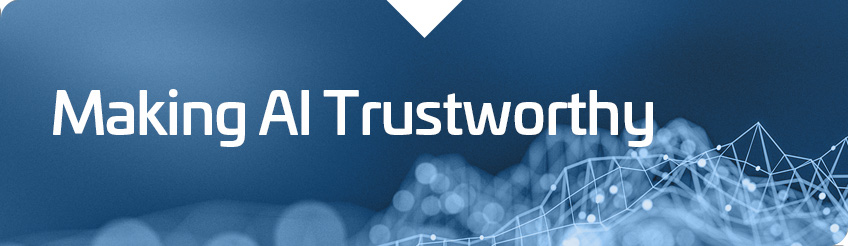
Decision making in high-stakes applications is increasingly supported by AI models, and there is a need to make them fair, robust, and trustworthy. In this seminar we will explore topics like:
- How fair are these models?
- Are the model decisions understandable and explainable?
- Can we build safeguards that prevent abuse and malicious behavior of AI models?
- How to build transparent reporting mechanisms on how AI models operate
Wednesday, October 23
Waterfront 1
7:30 am Registration Open
8:00 Continental Breakfast (Harborview Foyer)
9:00 – 12:00 pm AI World Executive Summit
12:00 Enjoy Lunch on Your Own
1:15 Making AI Trustworthy
Pin-Yu Chen, PhD, Chief Scientist, RPI-IBM AI Research Center, Research Staff Member, Trusted AI Group, IBM Thomas J. Watson Research
Center
This talk will include a holistic overview of IBM Research's portfolio toward trustworthy AI, including the dimensions of fairness, explainability, adversarial robustness and transparency. What you will learn in this presentation:
- Motivations and techniques for making AI models fair, explainable, robust, and transparent
- Advanced research for trustworthy AI
- Opensource libraries for trustworthy AI
1:55 Trusted AI for Advancing Science and Innovation
Payel Das, PhD, Research Staff Scientist and Manager, Deep Learning, AI Learning Department, IBM Thomas J. Watson Research Center; Adjunct
Associate Professor, APAM Department, Columbia University
Scientific discovery is one of primary factors underlying advancement of human race. However, traditional discovery process is slow compared to the growing need of new inventions, for example, new antibiotic discovery or design of next-generation energy
material. Data-driven approaches such as machine learning and, especially, deep learning have achieved remarkable performance in many domains including computer vision, speech recognition, audio synthesis, and natural language processing and generation
in recent years. Those methods have also infiltrated other fields of science including physics, chemistry, and medicine. Despite these successes and the potential to make huge societal impact, machine learning models are still at infancy in terms
of driving and transforming scientific discovery. In this talk, I will talk about a closed-loop paradigm to accelerate scientific discovery, which can seamlessly integrate machine learning, physics-based simulations, and wet lab experiments
and enable new hypothesis and/or artefact generation and validation thereof. Development and use of deep generative models and reinforcement learning-based methods for designing novel peptides and materials with desired functionality will be discussed.
Finally, I will discuss the importance of adding crucial aspects, e.g. creativity, robustness, and interpretability, to the machine learning models in order to enable and add value to AI-driven discovery.
2:35 Refreshment Break (Cityview Foyer & Harbor Level Atrium)
2:55 PANEL: Trusted AI
Moderator: Prasanna Sattigeri, Research, MIT-IBM Watson AI Lab, Thomas J. Watson Research Center
Panelists:
David Sontag, PhD, Associate Professor, Institute for Medical Engineering and Science (IMES); Principal
Investigator, Computer Science and Artificial Intelligence Laboratory (CSAIL)
Himabindu Lakkaraju, PhD, Post-Doctoral Researcher, Harvard University
Aleksander Mądry, PhD, Associate Professor, Computer Science; Principal Investigator, Computer Science and Artificial Intelligence Laboratory (CSAIL)
Elisa Celis, PhD, Assistant Professor, Statistics & Data Science, Yale University
- Fairness: Are our models fair? Unwanted bias and algorithmic fairness.
- Explainability: Are the model decisions understandable? Interpretability and causal inference.
- Robustness: Can our models be exploited? Safeguards that prevent abuse and malicious behavior of AI models.
- Assurance: How to build accountability into our AI systems? Transparent reporting mechanisms on how AI models operate.
4:10 Session Break
4:20 Plenary Keynote Panel (Harborview)
5:00 Grand Opening Reception in the Expo (Commonwealth Hall)
6:30 Attendee Roundtable Discussion & Meetup Groups
7:30 Close of Day